As you probably know, Java provides a comprehensive Collections framework. This framework defines lots of interfaces and classes for grouping objects and performing manipulations on groups such as insert, delete, sort and many more.
By using the interfaces provided we can write code less dependent on the implementation of the elements. In other words, we write decoupled code, which is really good, especially when you have to make changes to your application.
Another good thing about the interfaces defined in the framework is that they are generic — so we can instantiate a collection of any type we need (even a collection of collections).
Lists
One of the most used interfaces on the Collections framework is the List. A list is an ordered collection of elements which controls where in the list each element is inserted. This means that we are able to access an element inside a List by its position (index).
There are several types that implement the List interface, but the most used are ArrayList and LinkedList.
As both implement the same interface they do basically the same things, so in most cases, your code will work whichever implementation you use. But the performance of some operations will vary depending on the type of list you use. So the question we are going to try to answer is, which is better: ArrayList or LinkedList?
But before we discuss which implementation is better I would like to state that you should always avoid declaring a variable or a method as one of these types and instead use an interface.
Don’t
private ArrayList someList;
...
public ArrayList getMyList() {
ArrayList list = new ArrayList();
...
return list;
}
Do
private List someList;
...
public List getMyList() {
List list = new ArrayList<Integer();
…
return list;
}
So the short answer to the question “which implementation is better?” — as you’ve probably already guessed — is “it depends”.
So let’s analyze how these two structures work and find the advantages and disadvantages of each.
Specifically, let’s focus on the insertion (add an element at the end of the list), delete (remove an element from the list) and index operation (get an element by its index).
ArrayList
This implementation is the simplest of the two. It has an array under the hood which has a set capacity. Graphically, it looks something like this:
Inserting
This would be an empty list. If we think about the insertion operation, it is fairly simple, as the list keeps a variable for storing the size (number of the element in the list): we just have to insert the element in the cell with the index equal to the size. This is an O(1) operation (not really, but more on this later), which means that it runs in a constant time.
If you think about this structure, you are probably wondering what happens if we want to insert elements beyond the capacity of the inner array (in this case 6).
In that case, the insertion operation will have to do some extra effort: it will create a new array with more capacity and move all elements already inserted from one array to the other.
So, once in a while, an insertion operation will take longer than usual. This is why I said before that the complexity of the insertion operation wasn’t exactly O(1), it actually runs in amortized constant time, which means that when you are inserting large numbers of elements, only a small percentage of them will take longer, so the overall complexity will be equivalent to O(1).
Take a look at this answer in StackOverflow for more clarification about this.
Deleting
At first glance, it seems like a fairly simple operation, something that could run in constant time (O(1)), but it isn’t. Consider an array list with 5 elements:
If we want to delete the element in index 4, the last one, we just have to remove it and leave the cell empty. That’s all. But what happens if we want to remove the element in the index 0, the first one. We will end up with a ‘bubble’, an empty cell before the occupied cells. To avoid this, the array list will shift all the elements after the deleted element:
So, for this operation, the execution time will depend on the number of elements, because the more elements you have in the array, the more time it will take to shift them. That means that the algorithmic complexity for the deletion is O(n), which is not good at all.
Indexing
In this case, it is easy to see that the algorithmic complexity of this operation is O(1) for an array list. That means that it will take the same time to get an element by its index whether we have a hundred elements or a million.
Operation | Algorithmic complexity |
Inserting (at the last position) | O(1)* |
Deleting | O(n) |
Indexing | O(1) |
*amortized constant time
When we are working with lists, sometimes we know the exact size that our list is going to be, and sometimes we also know that the size will never change.
In such cases, we can do a little trick which consists of instantiating the array list with an initial capacity. This way we will avoid the case when you insert an element and you have to allocate more space.
Example:
public List myMethod(List originalList) {
List copyList = new ArrayList(originalList.size());
for(int i=0; i<originalList.size(); i++) {
Integer element = originalList.get(i);
element = element + 1;
copyList.add(element);
}
return copyList;
}
LinkedList
Java implements this class as a doubly linked list. This means that every element in the list have a reference to the next and the previous elements in the list. A graphical representation of this would look like this:
In this case, every element in the list will use more memory than in an array list as it has to hold two pointers, but it can be really useful for some cases.
Inserting
Inserting an element in a linked list means that we have to add a pointer in the previous last element of the list and another in the new element (to the previous last element).
Luckily the LinkedList also holds a couple of references to the first and the last element, so the operation only needs a few assignations, disregarding the number of elements in the list. This means that it runs in constant time so its algorithmic complexity is O(1), which, as you already know, is really good.
Deleting
In order to delete an element from a linked list, the first thing we have to do is to find the element, so we will have to traverse the list until we find it. We have to do that even if we already know the element’s position inside the list. If we want to remove the first or last element it will be much easier, as we have references to both elements, so the list provides special methods for deleting the last and first elements. But we are considering the general case in which you want to remove an element in a random position.
Once we’ve found the element in the list, it is fairly simple to remove it, we just have to make some assignations, which can run on constant time. Although, as you may guess, the algorithmic complexity for this operation will be O(n), the more elements we have in the list, the more it will take to delete one.
Indexing
As we have already seen in the deleting operation, finding an element by its index means that we have to traverse the list until we find it. So the operation of indexing is pretty similar to the deleting one. Thus its algorithmic complexity is also O(n).
Conclusion
Operation | ArrayList | LinkedList |
Inserting (at the last position) | O(1)* | O(1) |
Deleting | O(n) | O(n) |
Indexing | O(1) | O(n) |
*amortized constant time
In the table above we have the summary of the complexities of these operations for the array list and the linked list.
Next time you need to use a List in your code you can take these into account to choose between the two implementations. If you need a list for inserting elements at the last position and for getting them by its index then you should probably choose the ArrayList.
On the other hand, if you are going to perform more complex operations like inserting at the middle of the list or deleting from the head of the list (which we haven’t covered in this article) you probably want to use a LinkedList.
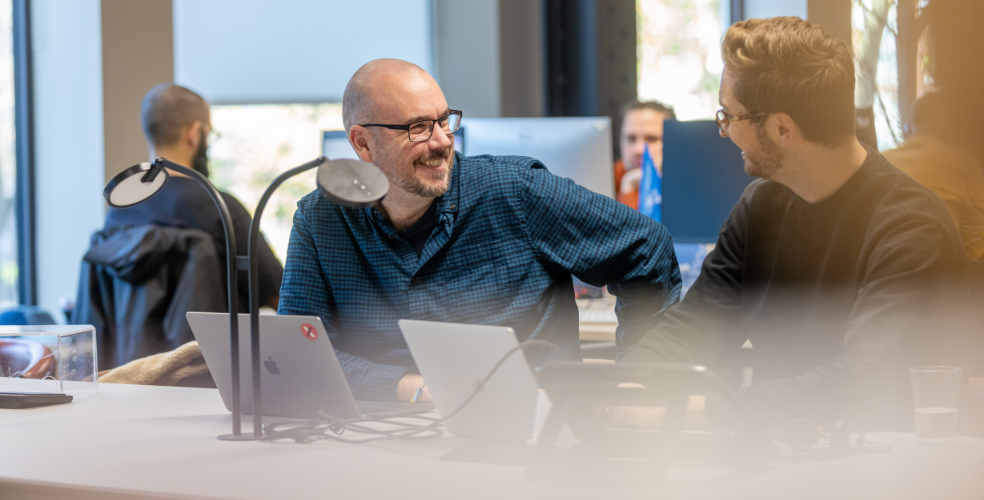
Transforming data sharing across government
We partnered with CDDO to pioneer a private beta working product that enabled cross government departments to share data.
Read moreOur recent insights
Transformation is for everyone. We love sharing our thoughts, approaches, learning and research all gained from the work we do.
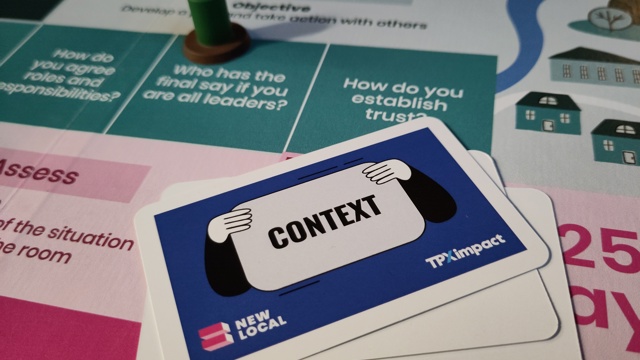
The role of play in building leadership skills
How play can offer local government leaders a powerful tool to break free from rigid structures.
Read more
A game-changing approach to leadership
Radical Leaders: The Game! uses real-world crisis scenarios to challenge local government leaders, fostering collaboration, agility, & community focus.
Read more
Transforming archiving through AI
How artificial intelligence can turn archives into living resources that shape the future while preserving the past.
Read more